Building Trust in Enterprise AI
Is AI ready for the demands of the business world? Explore the critical need for more transparency and trust in AI technologies and explore how the InstructLab project enables IT professionals to responsibly and ethically adopt these technologies within enterprise processes. From the risks of unknown training data to the promise of smaller, specialized models for enterprise applications, join us to look at how IT leaders can effectively deploy AI in today's ever-evolving business environment, both responsibly and ethically.
00:00 — Chris Wright
The breakneck pace of AI's evolution and adoption is sparking a rush to embrace a technology that still raises a lot of questions and concerns, because a single unchecked error can undermine years of building reputation and trust. The decisions we make about AI now are already shaping not just the future of individual enterprises, but the societal fabric as a whole. So it's imperative that we proceed with a strategy that prioritizes safety and reliability.
00:32 — Title Animation
00:39 — Chris Wright
Before we delve into the risks and drawbacks of integrating AI into our business operations, let's establish some context because without context there can be misunderstandings. Like in large language models, LLMs are trained on massive datasets gathered from the web, encompassing everything from scholarly articles and papers to social media posts. This vast array of data teaches them to understand and generate human-like text based on the patterns they detect, but that brings its own challenges.
01:14 — Prof Munmun De Choudhury
There are a lot of information on the internet, but not every information is accurate or credible. The biggest large language models are very smart AI, but they're not humans, they're not sentient, and because of that, when these models learn from all of this information on the internet, they're not just learning from accurate information. But they're also picking up miss and other forms of low quality information.
01:40 — Chris Wright
That's not to say that the largest LLMs aren't extremely good at what they do. In general, more parameters can lead to higher accuracy, but it becomes difficult to account for all the data that's going into your model. If some bad data makes it into your model, it's like a ticking time bomb. The model might operate smoothly until it doesn't, and that could lead to financial damage and reputational harm. But none of this takes into account the practicalities of general purpose LLMs.
02:12 — Akash Srivastava
If the model is too expensive to run, well, most enterprises will not be able to afford it. It's striking the right balance between the size of the model and the skill set or the knowledge that the model has, that can be tailored to the enterprise use cases is where the magic is.
02:25 — Chris Wright
The real promise of AI lies in smaller, specialized models crafted to meet precise business needs. These models aren't just more manageable, they're specifically designed to be cost-effective and highly functional within particular contexts. But that won't diminish the need for expertise and human oversight. AIs aren't exactly intelligent. They don't have ethics or accountability if we don't program those values in. So how do we align our AI with our human values?
03:06 — Akash Srivastava
Models are trained in phases. You pre-train them, then you align them. Alignment basically often means instruction tuning and preference tuning. Instruction. Tuning is when you're telling the model, Hey, learn to do this or memorize this. And then in preference tuning you tell the model, out of the five answers you gave for my question, I like this and this. And I want you to say those two answers when I ask the question next time.
03:36 — Chris Wright
But who should determine which responses we want from the model? We shouldn't expect data scientists to fully grasp the intricacies of fields outside their expertise when training AI. By empowering domain experts, we can enhance our model's relevance and accuracy without the complexities of requiring cross-disciplinary AI expertise.
03:58 — Akash Srivastava
The bar of contribution that customization of an LLM typically requires is very high. You have a lot of models. Some of them are open source, some of them you really know what the model was trained on. But if you want to take that model and customize it for your own use case, here's a set of things I want my model to know. Here's the list of things that I want my model to be doing, I call that prescriptive model building. InstructLab is a tool that allows you to prescribe what goes in your model and build a model that is truly made for your enterprise. What we have done with InstructLab is we have really decoupled the aspect where the prescription from an SME or a software developer that is required to customize the model is on one side. And then the real heavy lifting of how do you consume that prescription, convert that into a dataset, start the training that leverages multiple GPUs on the other side.
05:11 — Chris Wright
By knowing which data is going into our models and opening the development to involve diverse contributors in the testing and refining of models, we can gain collective expertise to accelerate innovation and build a foundation of reliability and trust.
05:27 — Akash Srivastava
All these testing requires a lot of people, a lot of manual effort. And that's what makes the building these models in the open source so much more appealing for doing AI the responsible way.
05:42 — Chris Wright
AI continues to be surrounded by uncertainty and debate, but projects like InstructLab are pioneering paths toward more open and trustworthy AI. It's a movement towards not just using AI but using it responsibly.
06:00 — Prof Munmun De Choudhury
I think that is probably one of the most important aspects of AI is making sure every person who can benefit or also get harmed by these technologies has a seat at the table. They have a voice, not just in being able to use these technologies in their work, in their businesses and in their lives, but also making sure that they can influence how we even design those technologies. And that's why open source AI is such a critical aspect of this whole field.
06:30 — Chris Wright
The real question isn't whether AI is a trend or a revolution. It's how we as leaders can deploy these technologies in a way that not only maximizes their utility, but also creates an AI that reflects our values and moves the needle in the right direction. Thanks for tuning in. We'll see you next time.
06:49 — CTA Screen
- Keywords:
- AI,
- ML
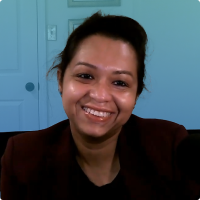
Dr. Munmun De Choudhury
Associate Professor, School of Interactive Computing
Georgia Tech
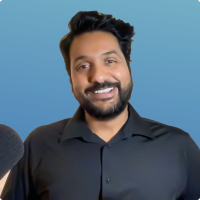
Akash Srivastava
Chief Architect, InstructLab
IBM
Keep exploring
What is InstructLab?
Find out how InstructLab empowers the enhancement of large language models in generative AI applications, with an approach that encourages broad community involvement, even for those without extensive machine learning experience.
What is AI/ML and why does it matter to your business?
It's vital to understand both the risks and benefits of Using AI effectively and ethically within your organization. Explore how Red Hat can help you foster trust in AI technologies and ensure they align with your values.
More like this

The role of the OS in the Age of AI
From supercomputers to cloud flexibility, learn about what’s shaping the future of operating systems in the age of artificial intelligence.
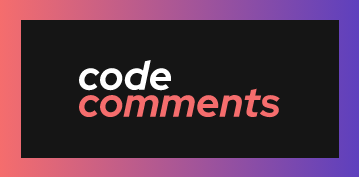
Bringing Deep Learning to Enterprise Applications
To realize the power of AI/ML in enterprise environments, users need an inference engine to run on their hardware. Two open toolkits from Intel do precisely that.
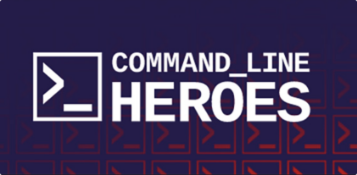
Talking to Machines: LISP and the Origins of AI
Like houseplants, machine learning models require some attention to thrive. That's where MLOps and ML pipelines come in.
Share our shows
We are working hard to bring you new stories, ideas, and insights. Reach out to us on social media, use our show hashtags, and follow us for updates and announcements.
Presented by Red Hat
Sharing knowledge has defined us from the beginning–ever since co-founder Marc Ewing became known as “the helpful guy in the red hat.” Head over to the Red Hat Blog for expert insights and epic stories from the world of enterprise tech.