Red Hat works closely with customers to provide the AI tools they need to build, deploy, monitor and use AI models and applications, but how do we utilize the power of AI for ourselves? Our Experience Engineering (XE) team is on it. The XE team collaborates with experts across Red Hat to deliver AI solutions to help our customers solve in-the-moment challenges via the Red Hat Customer Portal as we speak.
Troubleshooting recommendations
Troubleshooting recommendations are powered by a text-embedding AI model developed in collaboration with IBM Research, fine-tuned for re-ranking purposes. More specifically, it uses a custom IBM Slate Retriever model running in a Red Hat OpenShift AI cluster using GPU acceleration. The re-ranking feature combines very fast, traditional search with AI-powered textual analysis to more accurately order knowledge base solution recommendations. This enables users to locate the most relevant results faster and self-solve more effectively.
Automatic troubleshooting recommendations have been a function of the Red Hat Customer Portal for many years, but the new AI-powered update reorders the initial search results to present them in the optimal order based on the information provided by the user. Before the addition of AI-functionality, the first relevant article results users might see after initiating their search may have come up second, third or even 23rd depending on the number of solutions with similar words. Traditional search functions will return all the matches, while an AI-powered re-ranked search provides the most relevant results first. The more detail provided by the user, the more accurate the results!
After assessing the results, the customer can choose to view or ignore the recommendations, provide logs for additional automated analysis and decide if their question has been answered or progress through the user interface to open a support case with the already provided details. When a customer views at least one of the recommended solutions and doesn’t open a support case, we consider this as a successful self-solve engagement.
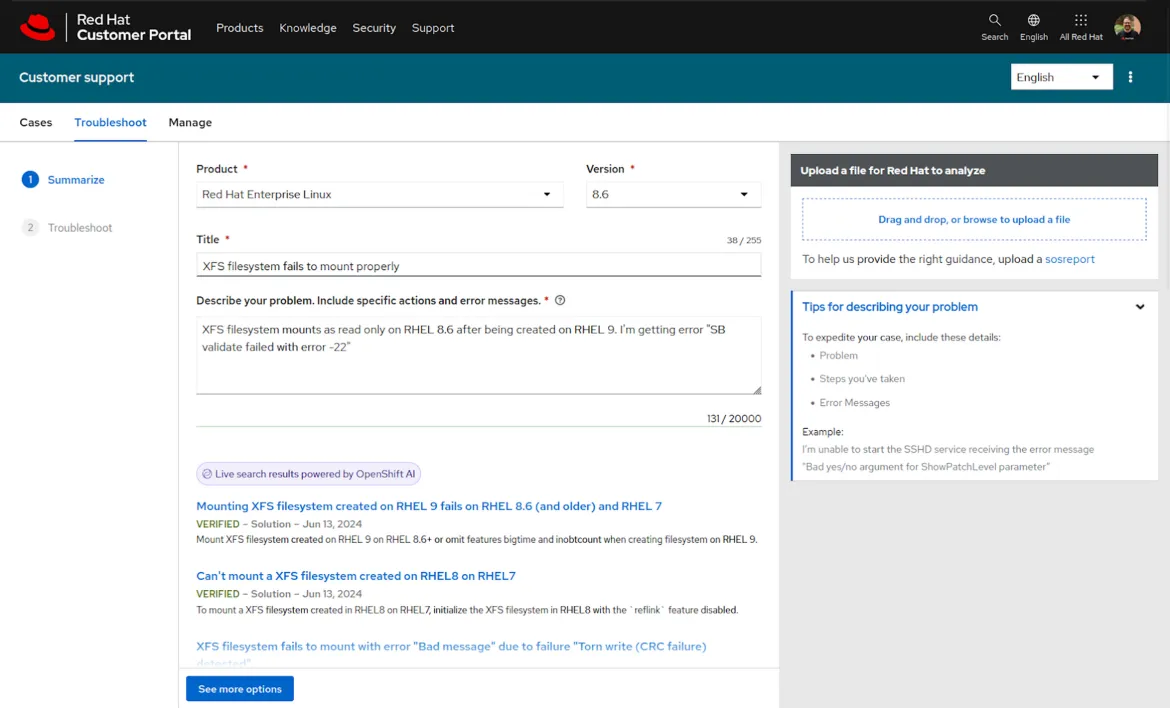
Red Hat’s XE team is focused on empowering customers with the option to self-solve issues with assistance from AI. This approach aligns with what our customers are looking for–a quicker and more effective resolution. With a greater capacity for self-solving common or lower-tier issues, the XE team is able to take the time and resources saved through the AI approach and apply them to creating solutions that address more nuanced or specific customer needs, with the intention to also bring AI to even more of these use cases in the future.
Knowledge base solutions summaries
Knowledge base summaries provide subtle, but impactful, assistance for finding and displaying search results. Powered by a generative AI summarization model also developed in collaboration with IBM Research, the feature currently uses Mixtral-8x7b-Instruct-v01 running within watsonx.ai on the IBM Cloud platform. The summarization API and update pipeline run in a Red Hat OpenShift AI cluster and utilize the OpenShift AI data science pipelines feature.
Historically, Red Hat knowledge base solutions did not have summaries or abstracts, which meant search and troubleshooting recommendation results could only display the first few sentences of the solution content, often only a restatement of the title or error strings. The lack of more useful context resulted in users having to click, open and view multiple solutions to find what they’re looking for. Each click meant additional effort from the user and delayed resolution to their problem.
This inspired the Red Hat XE team to develop a generative AI solution that creates and displays over 130,000 solution summaries, allowing customers to visually locate the most relevant solutions quicker and more effectively than before.
To accomplish this, the XE team collaborated with Red Hat knowledge management and content creation experts to review a subset of AI-generated summaries by comparing them to the traditional “first x characters” summaries. The results showed accuracy within a range of ~80% that the AI-Generated summaries were an improvement over the previous behavior. After releasing the feature, Red Hat support experts ran their own independent analysis of 200 solution summaries and found the AI-generated summary accuracy was as high as 94%.
This functionality, while slightly more challenging to measure, was a valuable stepping stone for the XE team and its collaborators in building new AI-generated summarization capabilities for the future. Since the release of these AI-powered customer-facing experiences, the team has seen a 20% increase in successful self-solve engagements compared to pre-AI engagements, which can quickly add up to real and impactful savings for a large-scale support organization like Red Hat’s.
You can see this functionality live on the Red Hat Customer Portal (authentication required) today. The Red Hat XE team continually strives to utilize our AI solutions to provide a better experience for our customers and improve how we help solve their challenges. We’re proud to showcase the capabilities of our AI platforms, as well as how we use them ourselves to build real world AI functionality using Red Hat products that directly impact and improve customer experience.
关于作者
Mandy is senior director, AI and Data, for Red Hat's Experience Engineering team.
Matt is the AI Portfolio Lead for Red Hat's Experience Engineering AI & Data team.
产品
工具
试用购买与出售
沟通
关于红帽
我们是世界领先的企业开源解决方案供应商,提供包括 Linux、云、容器和 Kubernetes。我们致力于提供经过安全强化的解决方案,从核心数据中心到网络边缘,让企业能够更轻松地跨平台和环境运营。